RAINER SCHOEBEL
University of Tuebingen - Faculty of Economics and Business Administration
JOCHEN VEITH
University of Tuebingen - Faculty of Economics and Business Administration
Inspired by the theory of social imitation (Weidlich 1970) and its adaptation to financial markets by the Coherent Market Hypothesis (Vaga 1990), we present a behavioral model of stock prices that supports the overreaction hypothesis. Using our dynamic stock price model, we develop a two factor general equilibrium model for pricing derivative securities. The two factors of our model are the stock price and a market polarization variable which determines the level of overreaction. We consider three kinds of market scenarios: Risk-neutral investors, representative Bernoulli investors and myopic Bernoulli investors. In case of the latter two, risk premia provide that herding as well as contrarian investor behaviour may be rationally explained and justified in equilibrium. Applying Monte Carlo methods, we examine the pricing of European call options. We show that option prices depend significantly on the level of overreaction, regardless of prevailing risk preferences: Downward overreaction leads to high option prices and upward overreaction results in low option prices.
Friday, June 27, 2008
Capital Market Theory: Is It Relevant to Practitioners?
David Nawrocki
Villanova University
A more complete theory is Vaga’s(1990) coherent market theory. Vaga argues that the market is constantly changing over time and is a time-varying process. As technology, investor expectations, government policy variables and rate of financialinnovation change, the market can experience different states -- steady state random walk, coherent cycles, and chaotic dynamics. Statistics may be used to describe Vaga’s market states. The random walk has stable symmetric (normal) distributions. The coherent cycle stage has stable skewed (nonnormal) distributions. The chaotic state has unstable skewed distributions. What are the implications? A stable probability distribution (random walk and coherent markets) will exhibit persistence over time. In other words, the distribution provides reasonable expectations of future performance. In addition, a coherent market is going to exhibit regularities that make the market forecastable with simple models. An unstable chaotic distribution implies that simple models cannot forecast the future.
Studying Vaga’s market states provides the interesting insight that his states relate to the business cycle. Vaga’s approach is statistical. However, the business cycle can be approached from an economics perspective. Hunt (1987) and Stovall (1996) have done interesting work on the stock market and the business cycle. Both authors divide the business cycle into different phases. We can divide the business cycle into four phases with each phase corresponding to one of Vaga’s market states. Four possible states of the business cycle are:
1. Easeoff -- The Federal Reserve is trying to cool down a rapidly growing economy that is experiencing increasing rates of inflation. The rate of economic growth slows and reaches a peak in economic activity. Interest rates peak.
2. Plunge -- The Federal Reserve’s anti-inflation policies cause a decline in economic activity (recession). A bottom (trough) occurs during this period. Interest rates and inflation decline during this period.
3. Revival -- The economy starts a recovery with strong economic growth, low inflation and low interest rates.
4. Accelerate -- The economy continues strong growth, however, capacity utilization starts to reach its limits and the economy overheats increasing the inflation rate.
Villanova University
A more complete theory is Vaga’s(1990) coherent market theory. Vaga argues that the market is constantly changing over time and is a time-varying process. As technology, investor expectations, government policy variables and rate of financialinnovation change, the market can experience different states -- steady state random walk, coherent cycles, and chaotic dynamics. Statistics may be used to describe Vaga’s market states. The random walk has stable symmetric (normal) distributions. The coherent cycle stage has stable skewed (nonnormal) distributions. The chaotic state has unstable skewed distributions. What are the implications? A stable probability distribution (random walk and coherent markets) will exhibit persistence over time. In other words, the distribution provides reasonable expectations of future performance. In addition, a coherent market is going to exhibit regularities that make the market forecastable with simple models. An unstable chaotic distribution implies that simple models cannot forecast the future.
Studying Vaga’s market states provides the interesting insight that his states relate to the business cycle. Vaga’s approach is statistical. However, the business cycle can be approached from an economics perspective. Hunt (1987) and Stovall (1996) have done interesting work on the stock market and the business cycle. Both authors divide the business cycle into different phases. We can divide the business cycle into four phases with each phase corresponding to one of Vaga’s market states. Four possible states of the business cycle are:
1. Easeoff -- The Federal Reserve is trying to cool down a rapidly growing economy that is experiencing increasing rates of inflation. The rate of economic growth slows and reaches a peak in economic activity. Interest rates peak.
2. Plunge -- The Federal Reserve’s anti-inflation policies cause a decline in economic activity (recession). A bottom (trough) occurs during this period. Interest rates and inflation decline during this period.
3. Revival -- The economy starts a recovery with strong economic growth, low inflation and low interest rates.
4. Accelerate -- The economy continues strong growth, however, capacity utilization starts to reach its limits and the economy overheats increasing the inflation rate.
An Evolutionary Quantum Game Model of Financial Market Dynamics - Theory and Evidence
Carlos Pedro Goncalves
Carlos Goncalves
The development of models that generate multifractal patterns in a bottom-up fashion is necessary, both for financial theorists and financial agents. The presence of multifractal patterns makes the markets more risky than predicted by standard financial models, which means that financial agents need to have models that are able to provide for guiding tools in asset and risk management.
Within standard financial theory the multifractal behavior remains unexplained. The development of a model capable of explaining the presence of multifractal signatures in the markets would be a first step towards a financial theory of market dynamics. However, such a model, and such a theory cannot be found in the framework of standard finance.
It has become necessary to review the microscopic assumptions that form the basis for classical finance, where, by microscopic assumptions, we mean the individual agent’s behavior and the interaction rules between agents.
Mathematical physics, and econophysics have provided for competing fields of research where it is possible to study market dynamics from the perspective of microscopic modelling. Spin glasses provide for the oldest examples of the application of physical models to solve unexplained empirical facts in market dynamics. One of the early applications of these models dates back to Vaga’s (1990) coherent market hypothesis, that tried to relate market dynamics to different phases, in an analogy with the different phases of a spin glass.
Although spin glasses have been an example of a simple and effective modelling tool to build microscopic theories of market dynamics, one still lacked a robust model capable of generating self-organized multifractality, until a recent work by Sornette and Zhou (Sornette and Zhou, 2005; Zhou and Sornette 2005, 2007), in which multifractal structure is diagnosed, not only by the standard convexity of the structure functions’ exponents, but also by a continuous spectrum of power law response functions to endogenous shocks.
Carlos Goncalves
The development of models that generate multifractal patterns in a bottom-up fashion is necessary, both for financial theorists and financial agents. The presence of multifractal patterns makes the markets more risky than predicted by standard financial models, which means that financial agents need to have models that are able to provide for guiding tools in asset and risk management.
Within standard financial theory the multifractal behavior remains unexplained. The development of a model capable of explaining the presence of multifractal signatures in the markets would be a first step towards a financial theory of market dynamics. However, such a model, and such a theory cannot be found in the framework of standard finance.
It has become necessary to review the microscopic assumptions that form the basis for classical finance, where, by microscopic assumptions, we mean the individual agent’s behavior and the interaction rules between agents.
Mathematical physics, and econophysics have provided for competing fields of research where it is possible to study market dynamics from the perspective of microscopic modelling. Spin glasses provide for the oldest examples of the application of physical models to solve unexplained empirical facts in market dynamics. One of the early applications of these models dates back to Vaga’s (1990) coherent market hypothesis, that tried to relate market dynamics to different phases, in an analogy with the different phases of a spin glass.
Although spin glasses have been an example of a simple and effective modelling tool to build microscopic theories of market dynamics, one still lacked a robust model capable of generating self-organized multifractality, until a recent work by Sornette and Zhou (Sornette and Zhou, 2005; Zhou and Sornette 2005, 2007), in which multifractal structure is diagnosed, not only by the standard convexity of the structure functions’ exponents, but also by a continuous spectrum of power law response functions to endogenous shocks.
Self-Organized Criticality in Synchronized Loss Dynamics - A Behavioral Account
Carlos Pedro S. Goncalves
ISCTE − Business School
Miguel A. Ferreira
ISCTE - Business School
Behavioral science and finance are the common element behind the different approaches to artificial financial markets. However, we can divide these approaches in two major branches, one is the branch that stems from a biological framework of analysis and, the other, is the branch that stems from a physical framework.
Although these two branches may be present in a given model, we usually find the presence of one or the other as dominant. For instance, the Santa Fe Artificial Financial Market combines behavioral finance and economics with genetic algorithms that are learning algorithms with a largely biological nature.
Goncalves (2004) artificial financial market, on the other hand, was largely influenced by the physical line of research. In particular, by Doyne Farmer’s work on agent-based modelling and econophysics, Sornette’s work in econophysics (in the modelling of financial crashes), Vaga’s coherent market hypothesis and the synergetics approach to complexity.
ISCTE − Business School
Miguel A. Ferreira
ISCTE - Business School
Behavioral science and finance are the common element behind the different approaches to artificial financial markets. However, we can divide these approaches in two major branches, one is the branch that stems from a biological framework of analysis and, the other, is the branch that stems from a physical framework.
Although these two branches may be present in a given model, we usually find the presence of one or the other as dominant. For instance, the Santa Fe Artificial Financial Market combines behavioral finance and economics with genetic algorithms that are learning algorithms with a largely biological nature.
Goncalves (2004) artificial financial market, on the other hand, was largely influenced by the physical line of research. In particular, by Doyne Farmer’s work on agent-based modelling and econophysics, Sornette’s work in econophysics (in the modelling of financial crashes), Vaga’s coherent market hypothesis and the synergetics approach to complexity.
Artificial Financial Market
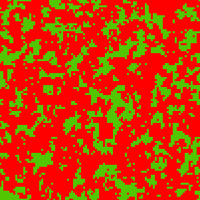
Carlos Pedro Goncalves
WHAT IS IT?
This is a model of an artificial financial market with heterogeneous boundedly rational agents that are influenced by the sentiment of their most close colleagues regarding the future evolution of the market.
The model is capable of generating the stylized facts of the real financial markets, specifically: excess volatility in the logarithmic returns, clustered volatility (characteristic of the well known GARCH signatures), bubbles and crashes.
The main influences behind this model were Vaga's coherent market hypothesis (Vaga, 1990) and Johansen, Ledoit, Sornette's model (Johansen et. al., 2002, Sornette, 2003), from now on denoted by JLS.
Our model may also be of interest to areas outside of finance, areas like, for instance, the study of social influence, opinion making and political decision.
Sunday, June 8, 2008
Oil Price Bubble?
For the past several years, there have been many who have suggested that oil prices may be rising in an unsustainable bubble. To be sure, there has been an upward bias in this market as reserve production capacity appears to have dwindled since around 2004. Major exporters such as Saudi Arabia have had production declines at a time when global demand has picked up with China and India enjoying rapid economic growth. The question of whether global oil supplies have peaked has enjoyed vigorous debate as prices have seen a dramatic rise over the past eight years.
The Oil Drum examined the question of whether oil prices were in a bubble back in 2006. Using Didier Sornette's approach of looking for faster than exponential growth in the time series of oil prices, this analysis by Stuart Staniford concluded that oil at the time did not exhibit the characteristics of past stock market or housing bubbles. He found that the logarithm (to base 2) of oil prices followed roughly a straight line:
"I have taken the start of the price rise as November 15th 2001 which is when prices bottomed out after the tech crash and the events of 9/11. To that price rise I fit both a linear trend, and a quadratic. To the extent the price was curving up in a bubblicious manner, we would expect the quadratic to depart markedly from the straight line. It elects not to do so - the two are very close. Thus we see that although there is considerable volatility in the price (and the pattern of that is worth further analysis in the future) the price rise is very much exponential in nature. So I take this as further evidence that we do not have a self-reinforcing bubble.
At least not yet."
So what has happened in the past two years since Stuart Staniford's analysis? Oil prices have continued to rise and recently has topped $128. On a log scale (base 2) this corresponds to 7. Is this just continuation of the exponential rise since 2001 or is there now more evidence of a faster than exponential rise which would suggest an oil price bubble that could be approaching a breaking point?
To look at this question, Figure 1 simply extends Staniford's analysis to the present time. The data is West Texas Intermediate from the same source, EIA. During the past two years the data shows first a correction in prices and then an accelerated rise to present levels. The past two years data represent a faster rate of rise than the prior period covered by Staniford's data. However, the linear fit to the log chart suggests that on average these may be random fluctuations in an overall exponential growth pattern versus the faster than exponential growth associated with a price bubble. The quadratic fit also doesn't deviate much from the linear fit over the period dating back to 2001.

Figure 1. Log (base 2) of West Texas Intermediate spot price in nominal US dollars together with linear and quadratic fits to the data. On this scale, 4 is $16, 5 is $32, and 6 is $64.
The next step is to extend the period of time covered by the exponential growth back to 1999 (approximately two years). This provides for the period that includes an initial price rise before the 2001 low. As shown in Figure 2 by including this period the exponential rate of rise is on average slower, leading to a much sharper contrast in the price action of the past two years. In other words, the linear fit to the entire time series has a lower overall slope and the sharp rise in prices the past two years is much greater than the overall exponential rise. The quadratic fit also deviates remarkably from the linear fit, again suggesting that there could be a possible faster than exponential price rise or bubble in the works.

Figure 2. West Texas crude spot prices from May 1999 to present.
Finally, we look for the possibility of an Log Periodic Power Law (LPPL) signature using Sornette's approach. The methodology to do so is based on fitting a somewhat complex LPPL function to the historical time series. This function has a faster than exponential characteristic and an oscillation that increases in frequency as the system approaches a singularity or critical breaking point. Unlike the linear or polynomial fits to historical time series, the LPPL method requires a guess as when the critical point will occur and fits the function back from this critical date.
Figure 3 presents one possible fit (not necessarily the best possible fit) of an LPPL signature to the oil price time series. It assumes a critical point at July 11, 2008. This is not a prediction of a crash on this particular date; rather it is used to illustrate the fact that there is a possible bubble with an LPPL signature. If the market continues its faster than exponential rise beyond this date, it would presumably be possible to revise the model to a new future critical date. Hence this methodology may be useful to indicate that the conditions for a bubble exist, though it may not provide a precise date for when the bubble might break.

Figure 3. Oil prices do exhibit the Log Periodic Power Law (LPPL) signature of a possible price bubble.
The Oil Drum examined the question of whether oil prices were in a bubble back in 2006. Using Didier Sornette's approach of looking for faster than exponential growth in the time series of oil prices, this analysis by Stuart Staniford concluded that oil at the time did not exhibit the characteristics of past stock market or housing bubbles. He found that the logarithm (to base 2) of oil prices followed roughly a straight line:
"I have taken the start of the price rise as November 15th 2001 which is when prices bottomed out after the tech crash and the events of 9/11. To that price rise I fit both a linear trend, and a quadratic. To the extent the price was curving up in a bubblicious manner, we would expect the quadratic to depart markedly from the straight line. It elects not to do so - the two are very close. Thus we see that although there is considerable volatility in the price (and the pattern of that is worth further analysis in the future) the price rise is very much exponential in nature. So I take this as further evidence that we do not have a self-reinforcing bubble.
At least not yet."
So what has happened in the past two years since Stuart Staniford's analysis? Oil prices have continued to rise and recently has topped $128. On a log scale (base 2) this corresponds to 7. Is this just continuation of the exponential rise since 2001 or is there now more evidence of a faster than exponential rise which would suggest an oil price bubble that could be approaching a breaking point?
To look at this question, Figure 1 simply extends Staniford's analysis to the present time. The data is West Texas Intermediate from the same source, EIA. During the past two years the data shows first a correction in prices and then an accelerated rise to present levels. The past two years data represent a faster rate of rise than the prior period covered by Staniford's data. However, the linear fit to the log chart suggests that on average these may be random fluctuations in an overall exponential growth pattern versus the faster than exponential growth associated with a price bubble. The quadratic fit also doesn't deviate much from the linear fit over the period dating back to 2001.

The next step is to extend the period of time covered by the exponential growth back to 1999 (approximately two years). This provides for the period that includes an initial price rise before the 2001 low. As shown in Figure 2 by including this period the exponential rate of rise is on average slower, leading to a much sharper contrast in the price action of the past two years. In other words, the linear fit to the entire time series has a lower overall slope and the sharp rise in prices the past two years is much greater than the overall exponential rise. The quadratic fit also deviates remarkably from the linear fit, again suggesting that there could be a possible faster than exponential price rise or bubble in the works.

Finally, we look for the possibility of an Log Periodic Power Law (LPPL) signature using Sornette's approach. The methodology to do so is based on fitting a somewhat complex LPPL function to the historical time series. This function has a faster than exponential characteristic and an oscillation that increases in frequency as the system approaches a singularity or critical breaking point. Unlike the linear or polynomial fits to historical time series, the LPPL method requires a guess as when the critical point will occur and fits the function back from this critical date.
Figure 3 presents one possible fit (not necessarily the best possible fit) of an LPPL signature to the oil price time series. It assumes a critical point at July 11, 2008. This is not a prediction of a crash on this particular date; rather it is used to illustrate the fact that there is a possible bubble with an LPPL signature. If the market continues its faster than exponential rise beyond this date, it would presumably be possible to revise the model to a new future critical date. Hence this methodology may be useful to indicate that the conditions for a bubble exist, though it may not provide a precise date for when the bubble might break.

Subscribe to:
Posts (Atom)